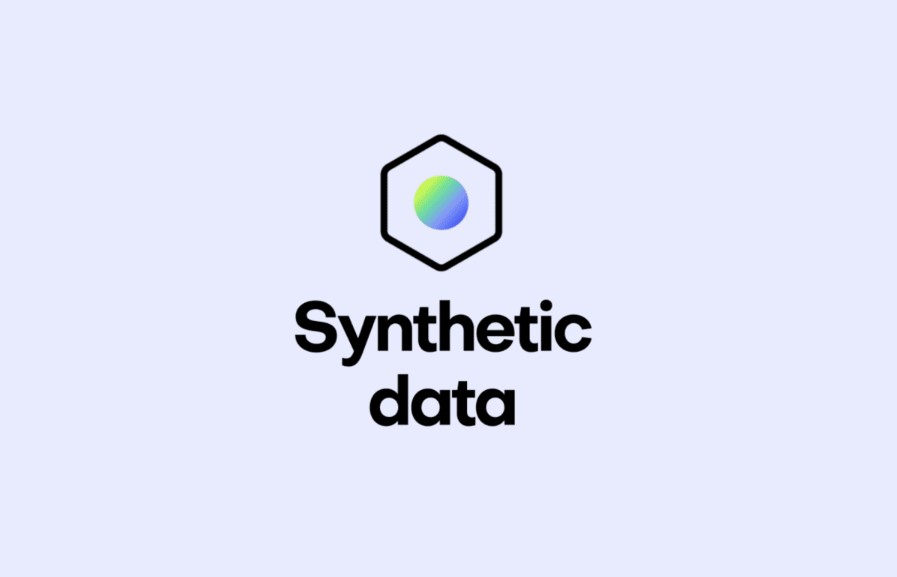
When was synthetic data invented?
The concept of synthetic data generation has been around for several decades, with its origins dating back to at least the mid-20th century. The specific techniques and methods for generating synthetic data have evolved over time alongside advancements in computing, statistics, and artificial intelligence. Here’s a brief overview of the historical development of synthetic data generation:
-
Early Statistical Methods: In the mid-20th century, statisticians and researchers developed early statistical methods for generating synthetic data. These methods often relied on random sampling, interpolation, and other mathematical techniques to create simulated data for statistical analysis and testing.
-
Simulation and Modeling: In fields like engineering, physics, and economics, computer simulations and mathematical models have long been used to generate synthetic data for studying complex systems and phenomena. These simulations aim to replicate real-world behaviors in controlled virtual environments.
-
Artificial Intelligence and Machine Learning: The advent of artificial intelligence and machine learning in the latter half of the 20th century brought about more sophisticated approaches to generating synthetic data. Generative models, such as neural networks and genetic algorithms, have been employed to create synthetic data that closely resembles real data.
-
Privacy-Preserving Techniques: With the rise of data privacy concerns and regulations in the 21st century, researchers and practitioners began developing privacy-preserving synthetic data generation methods. These methods aim to generate data that maintains statistical accuracy while protecting individual privacy.
-
Contemporary Developments: In recent years, deep learning techniques like Generative Adversarial Networks (GANs) and Variational Autoencoders (VAEs) have significantly advanced the field of synthetic data generation. These models have demonstrated the ability to generate highly realistic synthetic data, particularly in domains like computer vision and natural language processing.
While the concept of synthetic data generation has been in use for a long time, recent advancements in AI and machine learning have led to more sophisticated and effective methods for generating synthetic data. These methods have found applications in various industries, including healthcare, finance, autonomous vehicles, and more, as organizations seek ways to address data scarcity, privacy concerns, and the need for diverse datasets.